Unlocking the Mystery: An Insight into Explainable Artificial Intelligence (XAI)
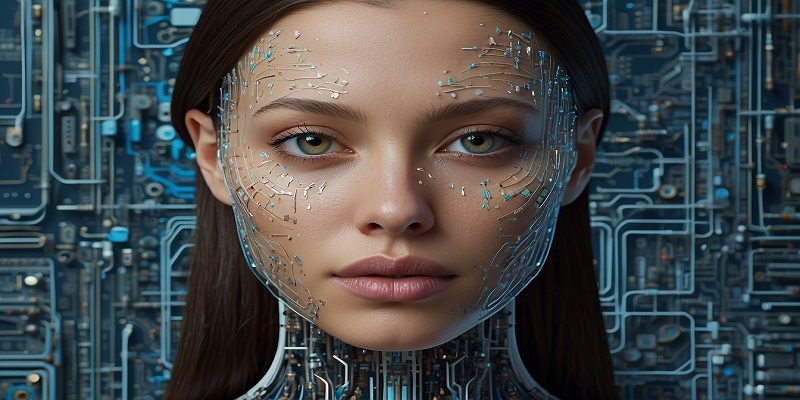
Introduction
In the realm of artificial intelligence (AI), there exists a fascinating concept known as Explainable Artificial Intelligence (XAI). Often referred to as the “black box” problem, the lack of transparency in traditional AI systems has raised concerns regarding their decision-making processes. However, with the emergence of XAI, a new era of understanding and transparency in AI has dawned.
Explainable Artificial Intelligence, or XAI, is a paradigm within the field of AI that focuses on creating systems capable of providing understandable explanations for their decisions and actions. Unlike traditional AI models, which operate as opaque “black boxes,” XAI algorithms aim to elucidate the reasoning behind their outputs, thereby enhancing transparency and trustworthiness.
The Importance of Transparency in AI
Transparency is paramount in AI systems, especially in applications where decisions impact human lives. Whether it’s in healthcare, finance, or criminal justice, stakeholders require insight into why AI systems make certain decisions. Without transparency, these systems can perpetuate bias, discrimination, and mistrust.
Challenges in Achieving Transparency
Despite its significance, achieving transparency in AI is no easy feat. One of the primary challenges stems from the complexity of modern AI algorithms. Deep learning models, for example, consist of millions of parameters, making it difficult to discern how they arrive at their conclusions. Additionally, proprietary algorithms developed by tech giants further exacerbate the lack of transparency, as companies are often reluctant to disclose their inner workings.
The Role of Explainability in AI Ethics
Ethical considerations play a pivotal role in the development and deployment of AI systems. By providing explanations for their decisions, XAI models empower users to evaluate the fairness and ethical implications of AI-driven outcomes. This transparency fosters accountability and enables stakeholders to identify and rectify biases inherent in the data or algorithms.
Applications of Explainable AI
Explainable AI has diverse applications across various industries, offering insights into decision-making processes and facilitating human-AI collaboration. In healthcare, XAI models can elucidate the factors influencing medical diagnoses, enabling clinicians to make more informed decisions. Likewise, in finance, explainable algorithms help investors understand the rationale behind investment recommendations, enhancing trust and accountability.
The Evolution of XAI Techniques
Over the years, researchers have developed various techniques to enhance the explainability of AI models. These techniques range from feature attribution methods, such as LIME (Local Interpretable Model-Agnostic Explanations), to model-specific approaches like attention mechanisms in neural networks. By leveraging these techniques, developers can design AI systems that not only perform effectively but also provide transparent explanations for their actions.
Future Directions and Challenges
While significant progress has been made in the field of XAI, challenges persist on the path towards achieving fully transparent AI systems. Technical hurdles, such as balancing model complexity with interpretability, remain a focal point for researchers. Moreover, addressing societal concerns regarding data privacy and algorithmic bias requires interdisciplinary collaboration and ethical considerations.
Conclusion
In conclusion, Explainable Artificial Intelligence represents a crucial step towards demystifying the inner workings of AI systems. By prioritizing transparency and accountability, XAI not only enhances trust in AI technologies but also fosters ethical decision-making across various domains. As we continue to unravel the complexities of AI, the pursuit of explainability remains paramount in ensuring a future where humans and machines coexist harmoniously.